If you look at a toothbrush on Amazon, you’re likely to be recommended toothpaste, mouthwash, and dental floss. This is a type of artificial intelligence called collaborative filtering that creates a graph of the relationship between products, even if they would seem to have no relation to each other.
For retailers to provide personalized experiences to their customers, being able to recommend the right product at the right moment is critical to staying competitive. Artificial intelligence is essential for this, as multivariate testing is essential for the modern marketer, and iterating on what works, while dropping ineffective creatives, keeps budgets in check for large retailers. Collaborative filtering is one of the most common AI approaches to solve the issues presented to a recommendation engine. A good way to think of collaborative filtering is that the engine pays attention to product attribute over people attributes. Consumers are an intermediary node in the computation, rather than a feature of the graph. Both the input and output are the product attributes.
However, collaborative filtering does have its limitations. Consider a product graph that predicts dental floss, mouthwash, and toothpaste because a user browses for a toothbrush . Meanwhile, a hairbrush predicts a comb, shampoo, and conditioner. Does that mean that a consumer who considered purchasing both a toothbrush and a hairbrush is best served by recommendations for dental floss, mouthwash, toothpaste, comb, shampoo, and conditioner?
Perhaps. But to be thorough, it’s necessary to look into the possibility that there may be something special about having an interest in both of these products in addition to other observed data like seasonality. Consider the back to school period and add the demographic data of college student to the profile. Suddenly, if you are a retailer, you might be considering far more interesting items to add to the recommendations list. This consumer could be stocking up on all types of college supplies, and therefore may also be interested in a fresh wardrobe, bedding, notebooks, and so on. Simply put, only a model that preserves people as both an input and an output can hope to make better predictions in a multi-choice world.
An even more important requirement is the need to offer recommendations to consumers who have no shopping history. In this situation, an individual consumer has not expressed any product intent, which makes a recommendation difficult. Instead, the recommendation is driven by the resemblance of the user to a certain group of people.
For example, a retailer may have discerned that stay-at-home mothers in their early 40s are more likely to buy laundry detergent, pet food, and plant fertilizer than other target segments. If a consumer with no shopping history appears to fall into that demographic, predicting that she wants to purchase those three items seems to be the best you can do.
Although much more computationally expensive, neural network technology can use the attributes of these individual people to make predictions, rather than relying on shopping history alone. The benefit of being able to make a prediction based on who you are, rather than just the products that may or may not match your interests, can pay dividends for retailers.
ad personalization,
Marketing Creative,
innovative digital marketing,
online advertising,
trends,
retail
|
January 25, 2019
|
By Serge Ioffe
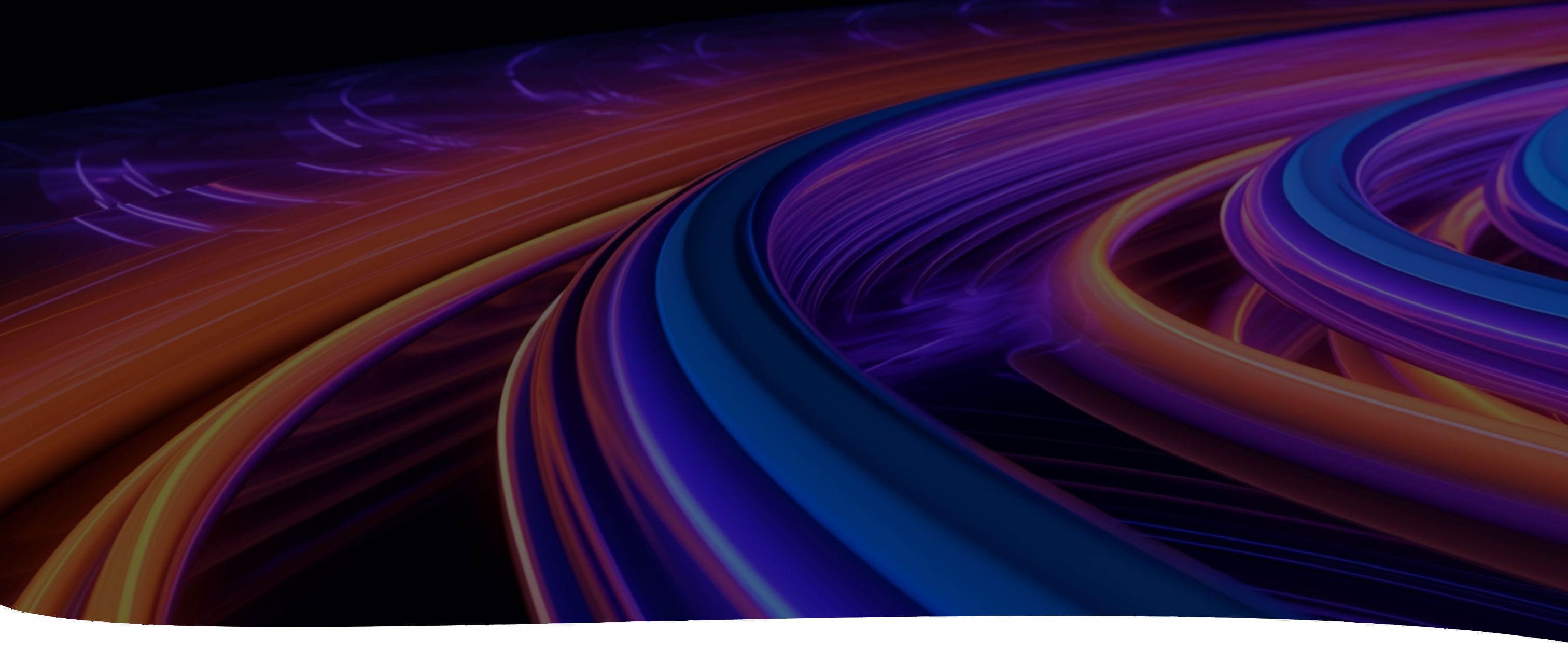